Navigating the Future of Edge AI: Observability and Intelligent Data Selection
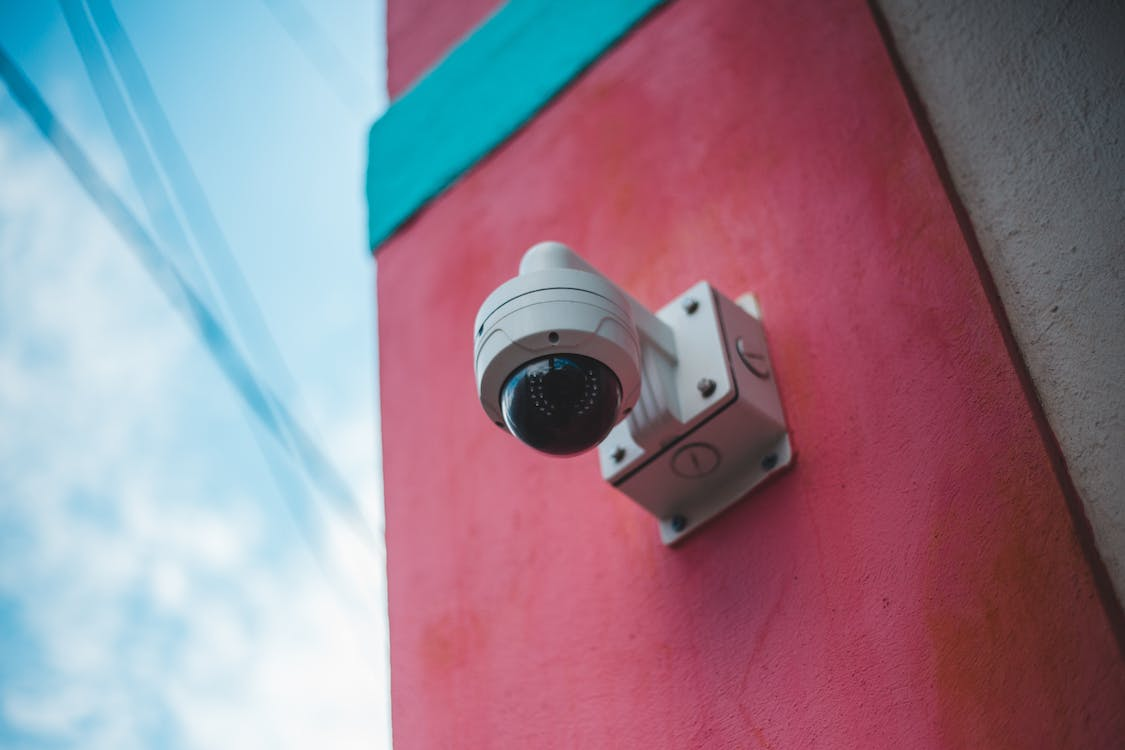
The Lightly team recently had the pleasure of attending the 2023 AI Hardware & Edge AI Summit, immersing ourselves in groundbreaking discussions and sharing insights with pioneers in the field of Edge AI. Through all the innovation and challenges, Pushpak Pujari’s statement from Verkada cut through the noise:
“AI Observability on the edge is still unsolved.”
Back to the Cloud? Not for Everyone
While Large Language Models (LLMs) are witnessing a significant shift back to the cloud, computer vision, a predominant application for running AI on the edge, treads a different path. The sheer volume of data involved is too extensive, rendering cloud-based inference impractical. Consequently, a growing number of companies are advancing towards edge-based solutions, addressing the inherent challenges in building Edge AI applications.
The Practical Challenges
Building Edge AI is hard. It’s not just about deploying models; it’s about managing them in the wild. Pushpak laid out the three big headaches during his presentation:
- Data
- Deployment
- Drift
Data: It's About Reality
For models to work on the edge, they need to be trained with datasets that mirror the real world to avoid unexpected breakdowns in production.
The data should represent various conditions and scenarios, and bias should be minimized. It’s imperative that datasets contain diverse sites, angles, objects, scenes, lighting, and weather conditions, minimizing the risk of failure due to unanticipated variables. Data bias goes beyond mere representation and encompasses data redundancy that can hurt model performance. Organizations must either construct internal data curation tools or leverage external ones to mitigate these issues.
Deployment: It's a Balancing Act
Deployment is critical and tricky. It needs to work on different hardware and requires regular updates. Managing a large number of devices is already challenging, and AI adds another layer of complexity to it.
Ideally, updates can be done over-the-air (OTA). Managing extensive fleets of devices is already a complex task, fortunately, mitigated by tools like Mender or Balena. However, introducing AI further complicates the situation, especially regarding MLOps and data pipeline management. Data must flow bidirectionally while maintaining stringent data privacy standards.
Data Drift: Keep Up or Fall Behind
Data drift is a constant challenge for companies deploying on the edge. Deployed models need to adapt to new environments and situations, requiring the integration of new data into the training sets to maintain their efficiency and accuracy.
Regular deployments in diverse locations necessitate the incorporation of environmental data into training sets, ensuring model resilience and generalization. This requirement introduces challenges related to data transfer volumes, labeling costs, and increased compute costs due to expanding training datasets. However, these tasks are crucial to maintain optimal model performance and prevent data or model drift in production environments.
How Can Data Curation Help?
Data curation using techniques like over- or undersampling and active learning can optimize datasets, improve model performance, and reduce training set sizes. A data curation tool on the edge can monitor live data streams and select valuable data in real-time.
Data curation plays a significant role in mitigating all the above-mentioned challenges. It enhances dataset quality and enables the identification and selection of valuable data, optimizing model performance in terms of generalization and robustness. Implementing a data curation solution on the edge serves as a powerful tool to monitor live data streams continuously for anomalies or out-of-distribution data.
The Outcome?
Implementing data selection and observability on the edge can lead to:
- Reduced compute costs due to optimized training set sizes
- Lower labeling costs due to efficient data selection
- Minimized transfer & storage costs due to selective data on the edge
- Better model performance due to high-quality training data
- Early detection and rectification of data drift and model drift through edge observability and selection
Lightly’s Solution
Lightly offers a data curation solution powered by active learning, providing observability and data selection on the edge to enhance AI. If you want to optimize your AI, connect with us.
Matthias Heller
Co-founder Lightly.ai
Thanks Laura Schweiger, Malte Ebner, and Igor Susmelj for reviewing drafts of this blog.