Build state-of-the-art ML Pipelines
with Active Learning
Lightly selects the subset of your data with the biggest impact on model accuracy, allowing you to improve your model iteratively by using the best data for retraining.
Build state-of-the-art ML Pipelines
with Active Learning
Lightly selects the subset of your data with the biggest impact on model accuracy, allowing you to improve your model iteratively by using the best data for retraining.
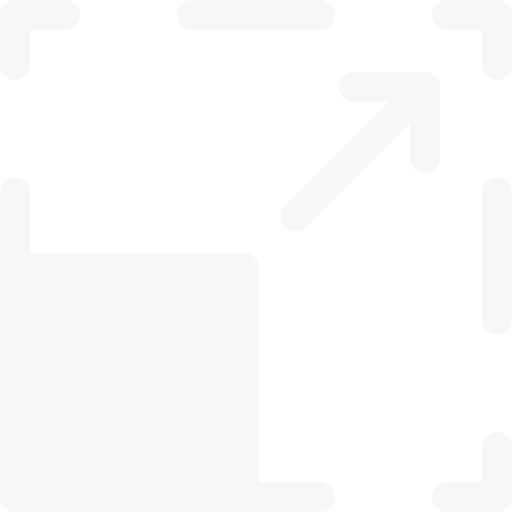
Scale
Select the best 1% from millions of images or videos
Lightly's algorithms can process lots of data (e.g., 10k videos or 10m images) within less than 24 hrs
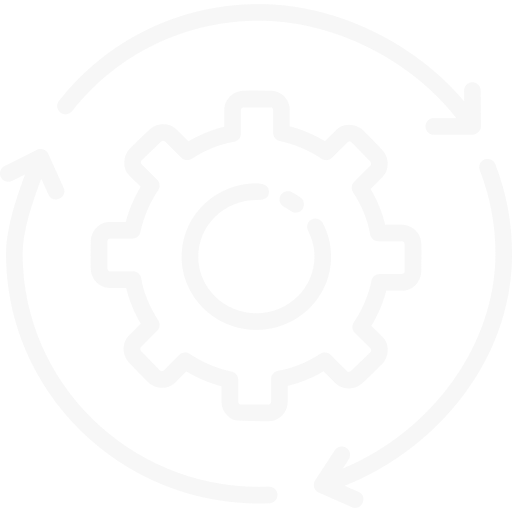
Automation
Use our API to automate the whole data selection process
Connect Lightly to your existing Cloud buckets and process new data automatically
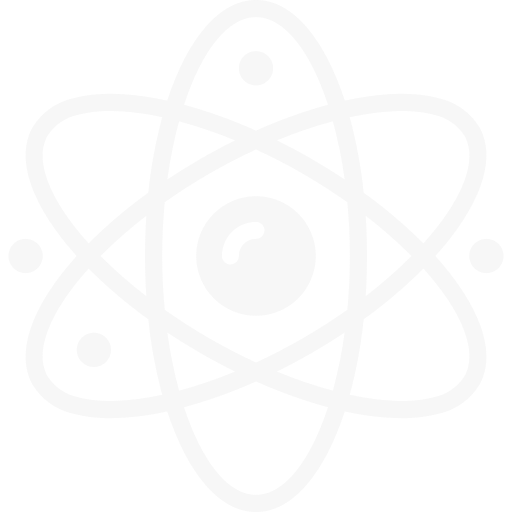
Science
Use state-of-the art active learning algorithms
Lightly combines active- and self-supervised learning algorithms for data selection
Features that lift you to the next level
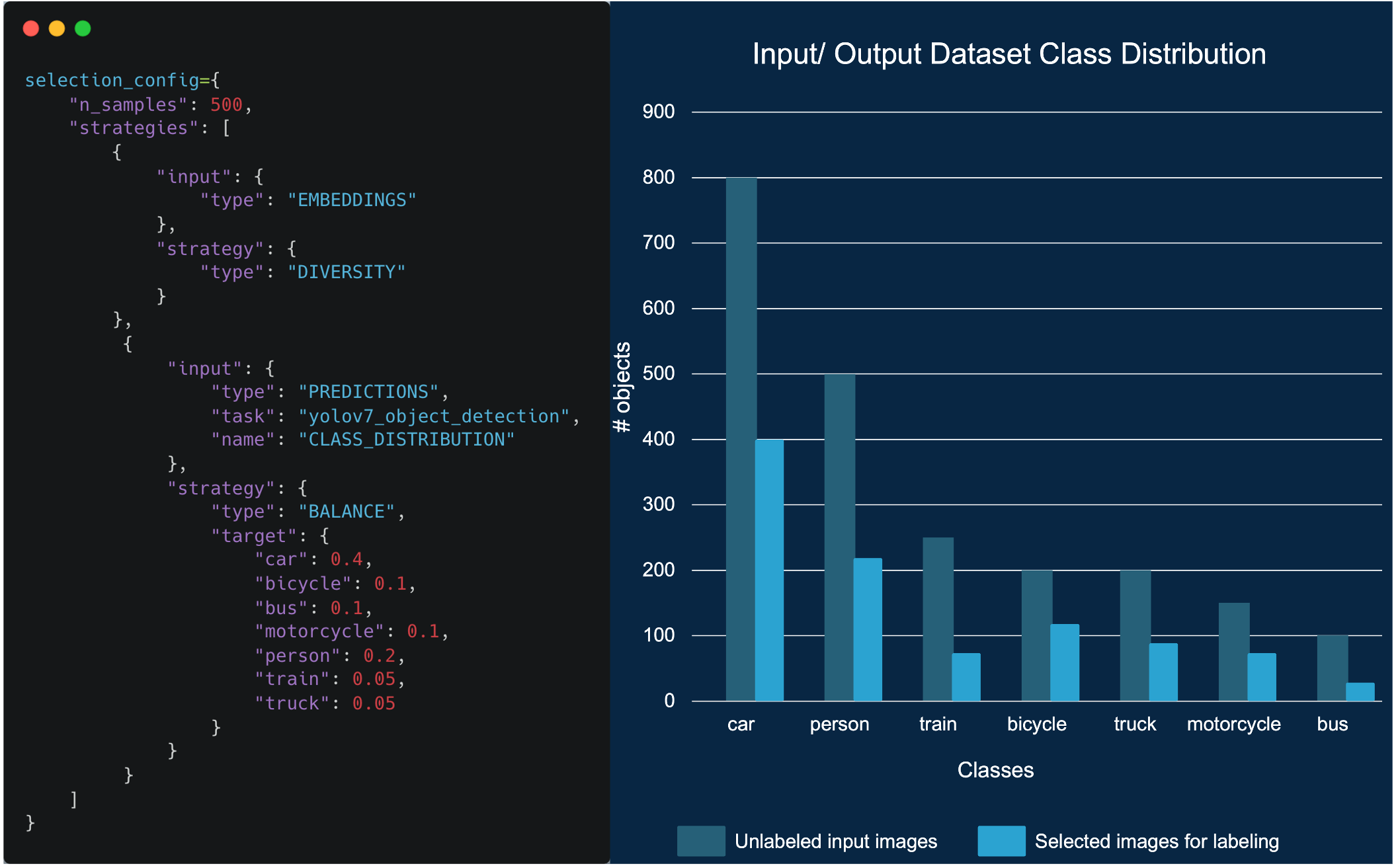
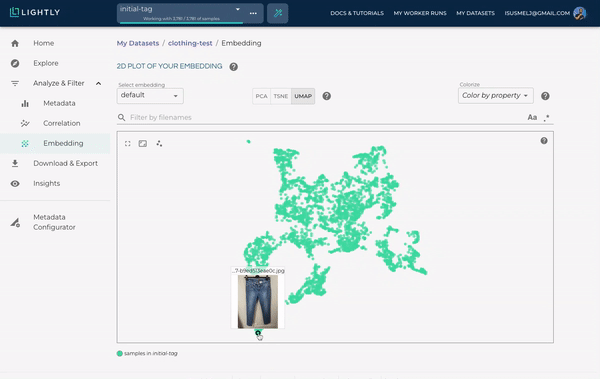
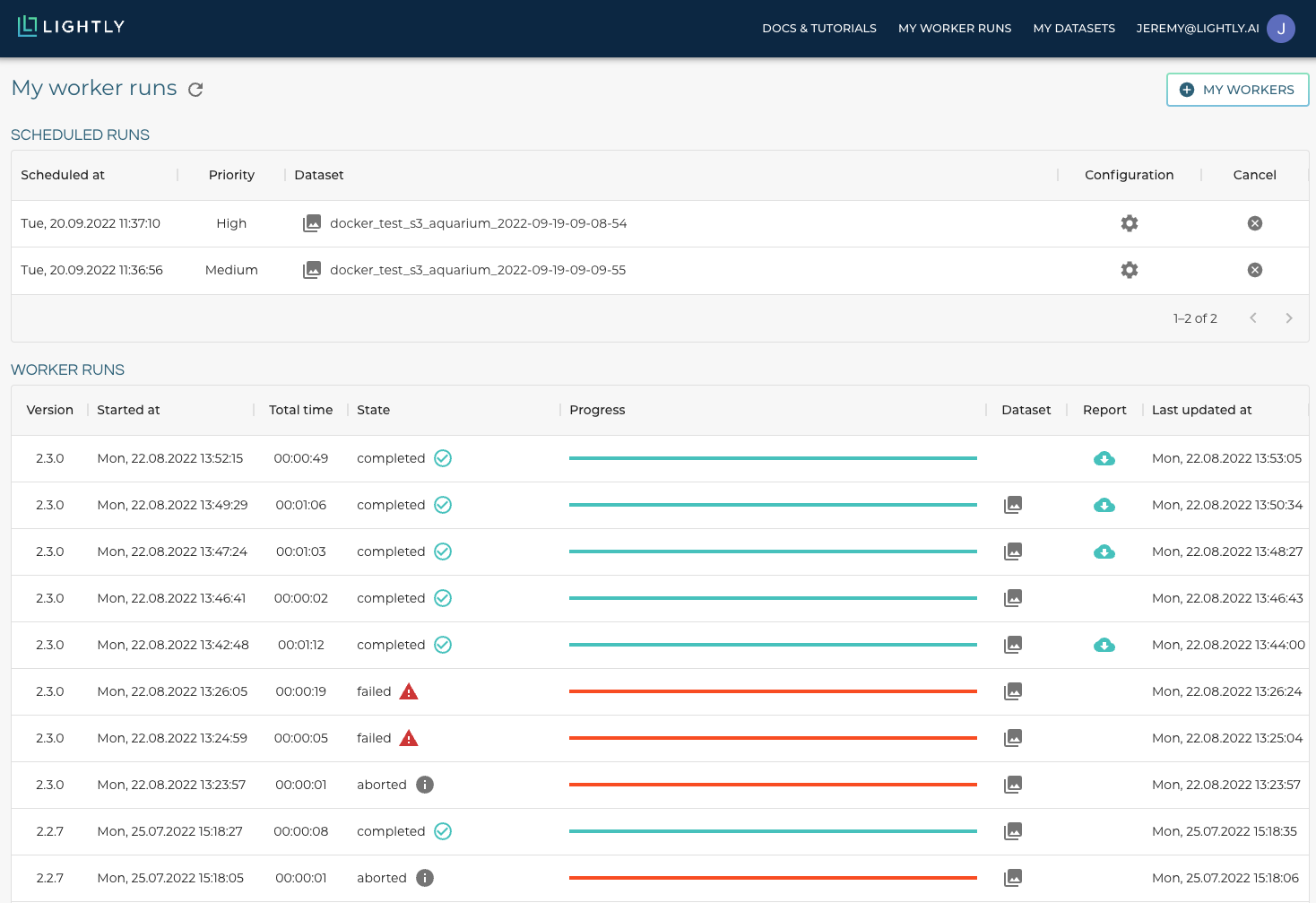
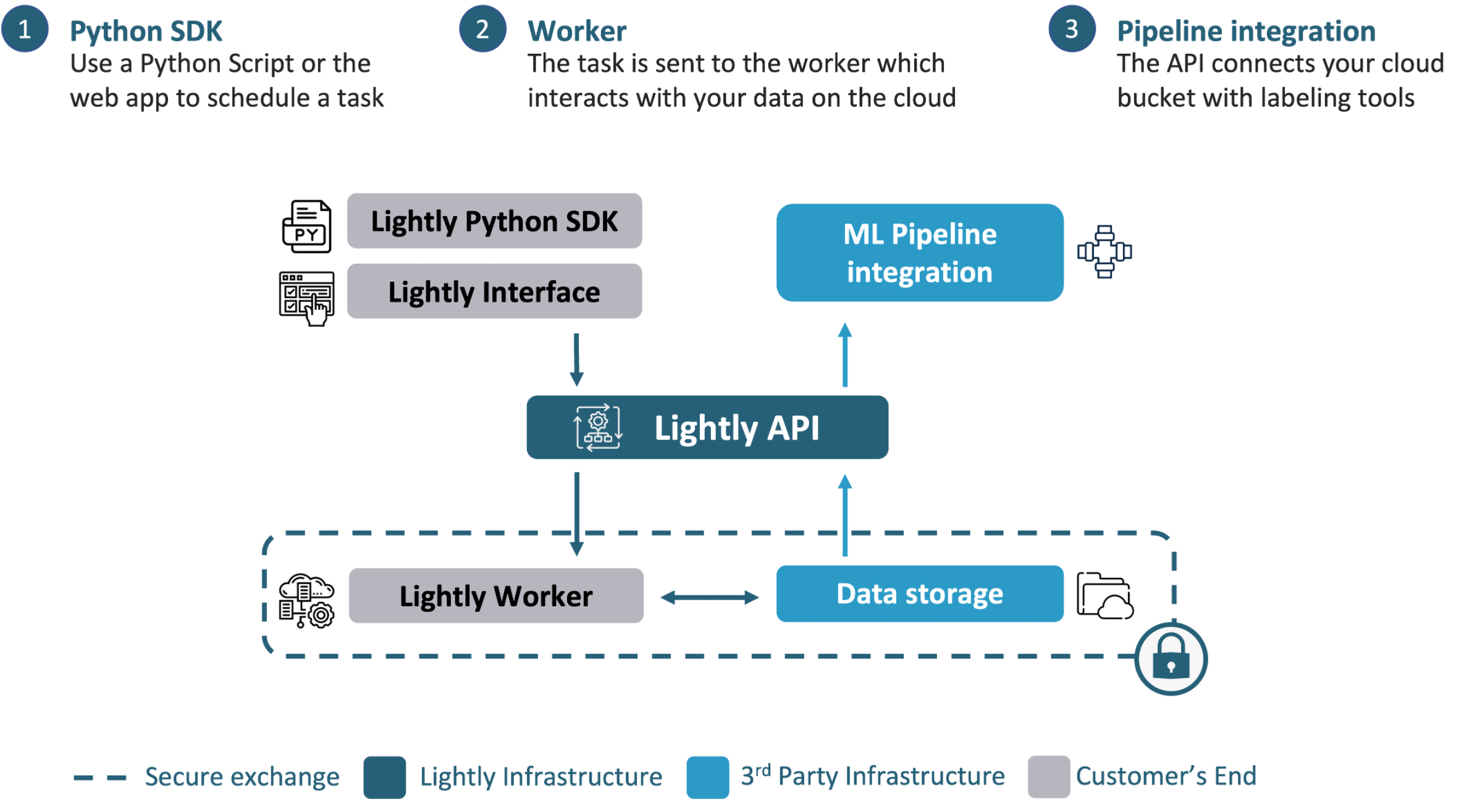
We help customers to have up to
90%
less labeling costs
Data redundancy can not only hurt model performance but also create significant costs for data labeling, storage, and compute. Don't waste your money on bad data!
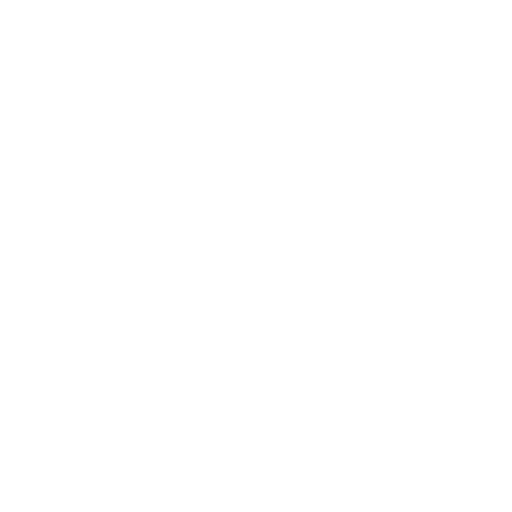
- Tired of only selecting 1 frame per minute to reduce the data load?
- Want to get randomness out your data?
- Manually picking doesn't give you the best result?
Lightly will be able to help you with that and will make selecting the best data easy for you
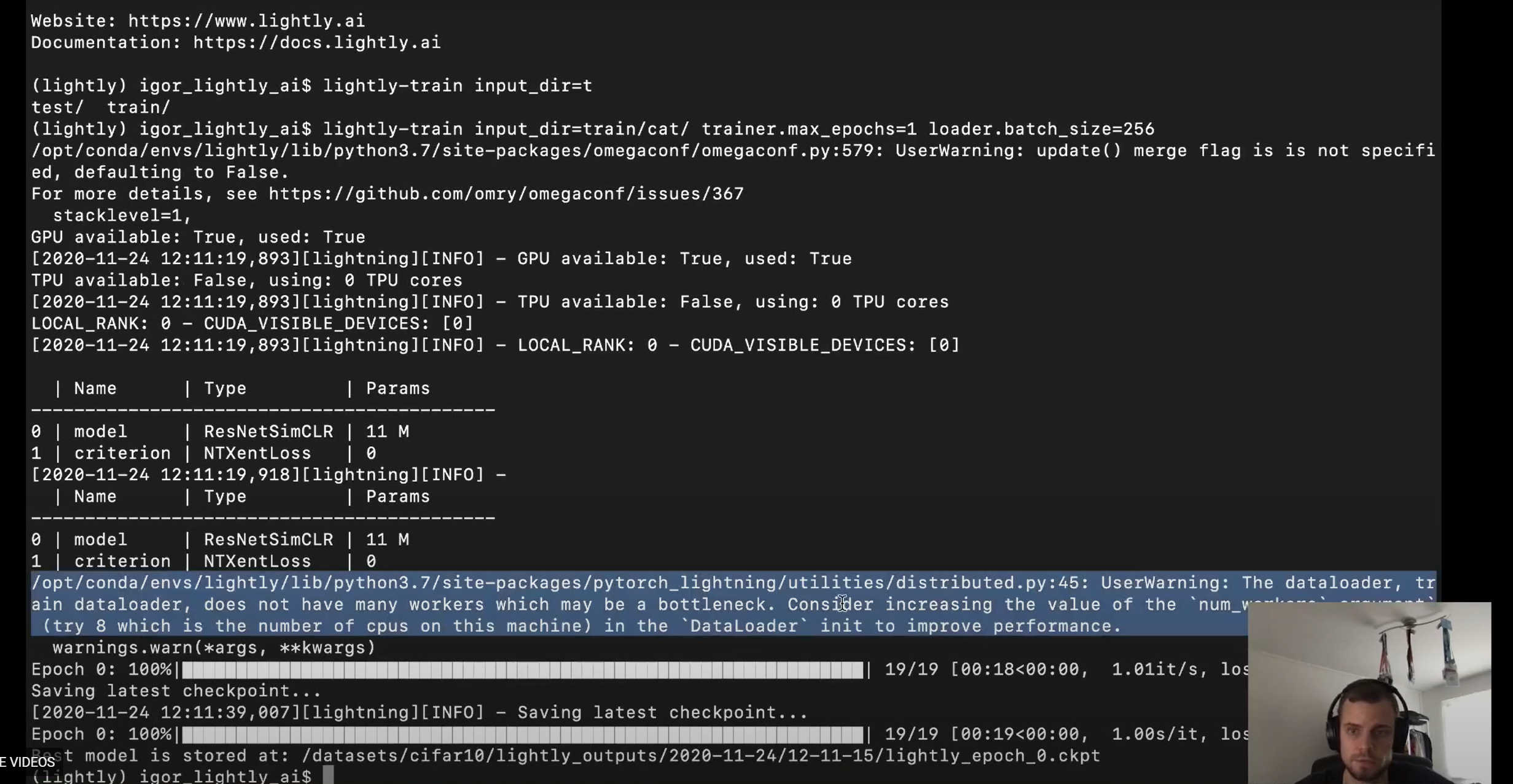
20%
better models
Selecting training data that is difficult for your model can yield significant gains in accuracy. Use active- and self-supervised learning approaches thanks to Lightly.
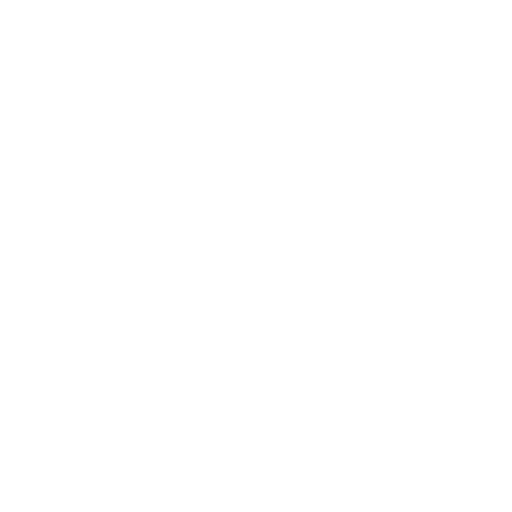
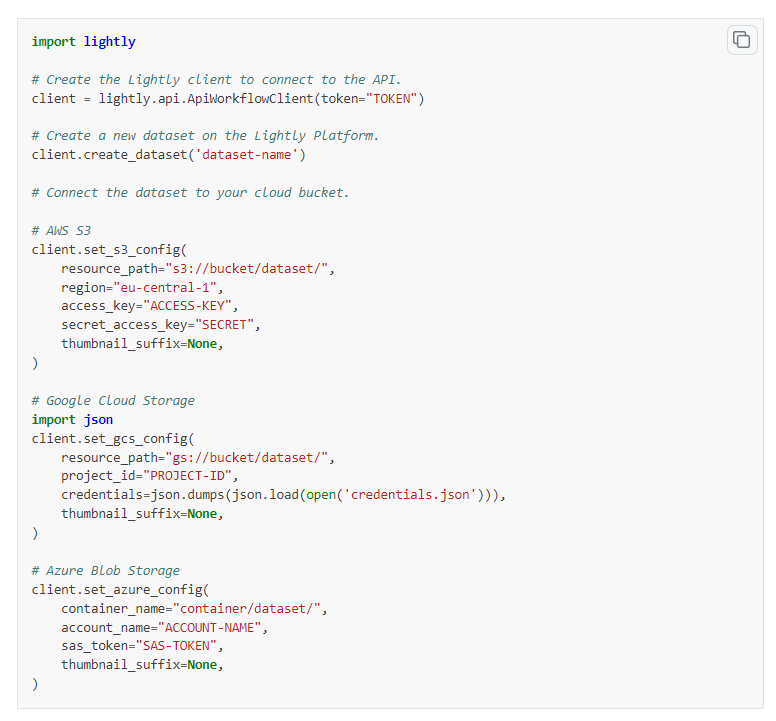
- Send the best subset of your data to labeling at the click of a button
- Trigger retraining and model deployment
- Automatically build datapools and datasets
2x
faster retraining cycles
Managing your data and machine learning pipeline efficiently saves a lot of time and reduces errors. Leave hacky in-house solutions and scripts behind for a scalable and reliable solution.
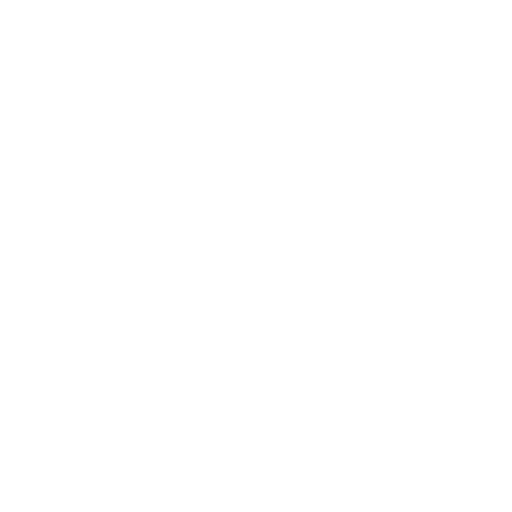
- Send the best subset of your data to labeling at the click of a button
- Trigger retraining and model deployment
- Automatically build datapools and datasets
- Take the human error factor out of your data curation equation
- Get access to cutting-edge data curation technology
- No need to implement research papers yourself
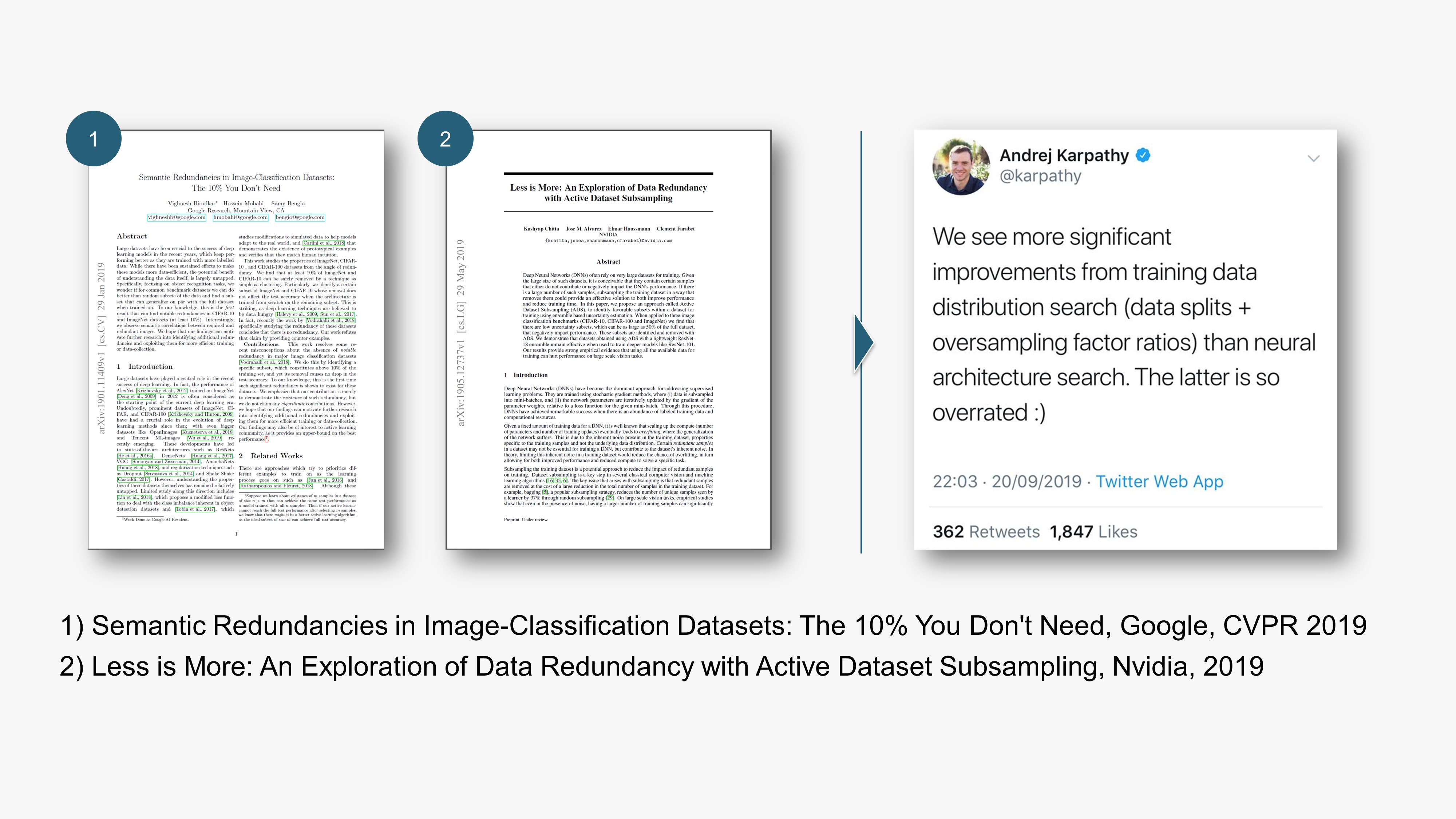
We trust in hard math and base Lightly on it
Ask our customers
4 easy steps to configure your ML pipeline
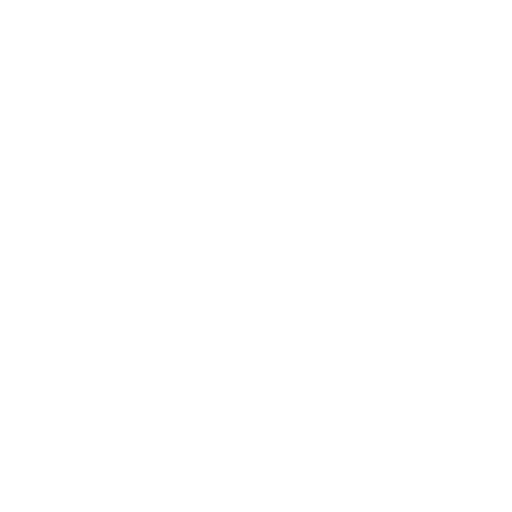
Connect
Connect Lightly with your data in GCP, Azure, and AWS S3 buckets. Data stays on your infrastructure, which keeps your it secured
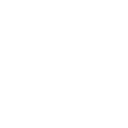
Configure
Use a combination of model predictions, embeddings, and metadata to reach your desired data distribution
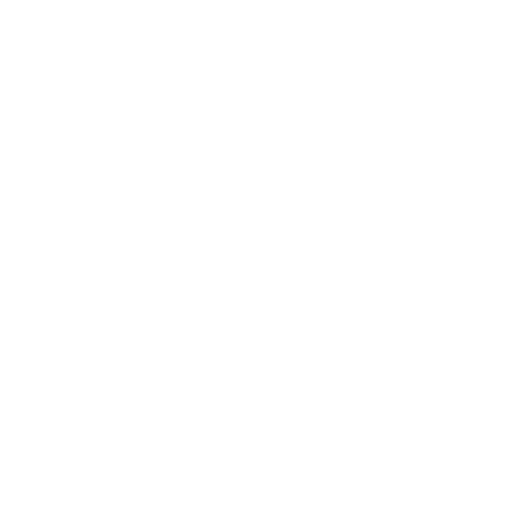
Run
Process data on your infrastructure using a docker container. Our solution streams data from the bucket without cluttering disks
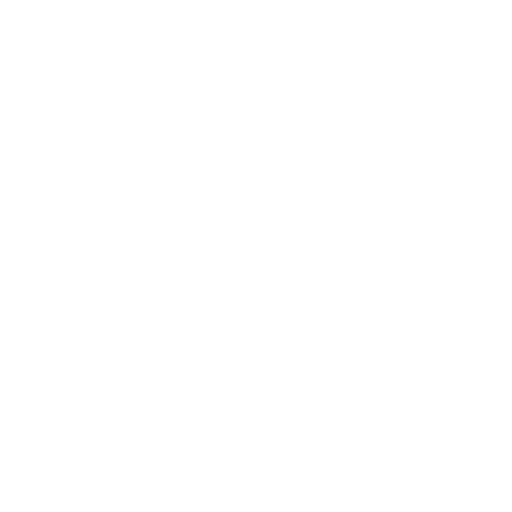
Use
Get your curated dataset labeled, train your machine learning model, and check the accuracy improvement
Integrate with your ML Stack
Designed to seamlessly plug into your favorite storage, tooling, and service providers in order to build an automated data pipeline for machine learning that enables a closed loop feedback cycle.
Data Storage
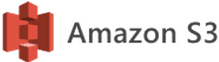

Label Tooling


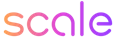
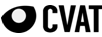
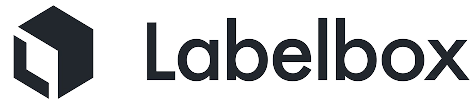
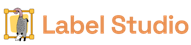
Model tooling
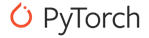

