Sustainable AI and the New Data Pipeline
Data, data, and more data. Do you have a question? Ask the data for an answer. Couldn’t find an answer? You just need more data, or do you?
Since the rise in popularity of deep learning, corporations and governments have gathered as much data as is feasible. The hope is for deep learning to automate anything and predict everything else, as long as data is supplied in large enough quantities. This paradigm has brought an exponential increase in collected data. Indeed, as Figure 1 shows, the volume of data created worldwide is estimated to increase by more than 200% every five years. However, the precondition of increasingly larger amounts of data might challenge the validity and legitimacy of deep learning as we advance.
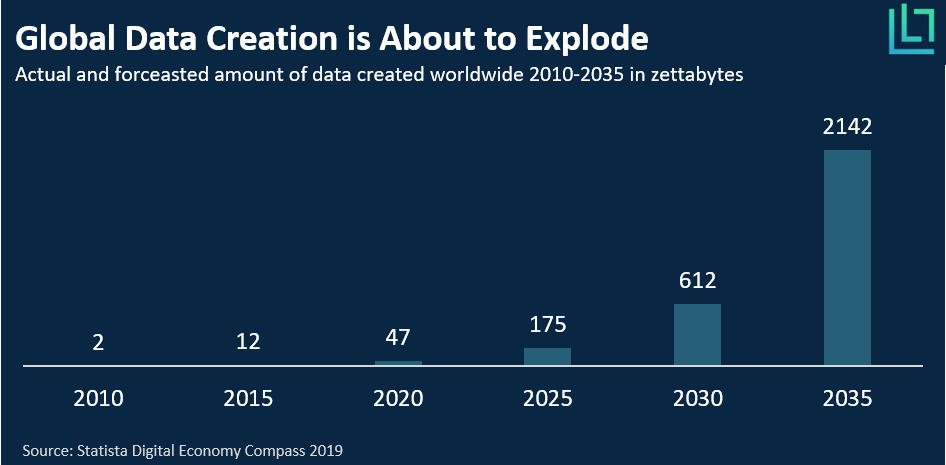
Challenging Deep Learning and Sustainable AI
But don’t get me wrong here. Deep learning has a plethora of remarkable and sometimes disquieting applications. Indeed, deep learning allowed for tremendous progress in autonomous driving, NLP, healthcare, and many other fields. However, current ML algorithms suffer from low interpretability and depend on large amounts of data. So much so, that research shows that training models produces significant quantities of CO2 due to the required computing power. As Figure 2 illustrates, training a relatively large model consumes as much as four cars do during their average lifetime. Therefore, the growth in required data and computing power raises questions of whether AI is sustainable.
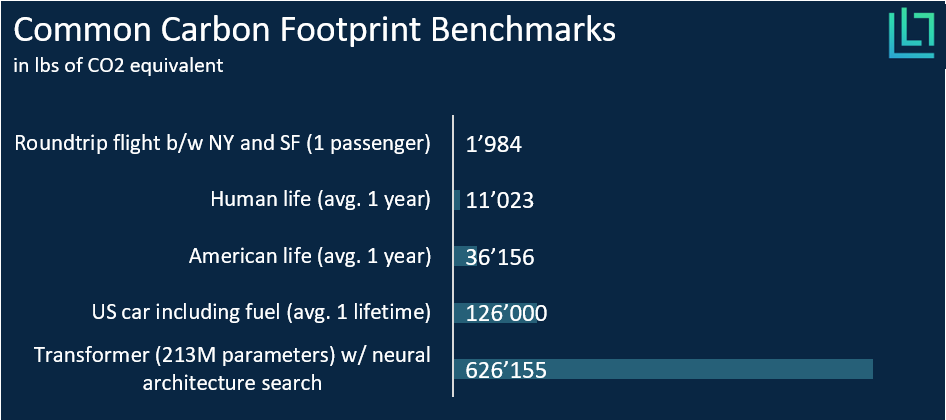
Moving on from computing power, another issue with deep learning resides in the growth of new data being driven mostly by unstructured data. However, classic deep learning models require structured and labeled data to be trained. The issue then becomes obtaining structured data from the unstructured, which almost always requires human labor. Given the repetitive nature of such work, large companies commission cheap labor in developing countries, which, depending on the labor conditions, can be viewed as a dubious practice.
Furthermore, assuming the same growth for unstructured data and general data, the demand for labeling services will be thirteen times greater by 2030 than it is today. Ceteris paribus, the price per label will increase accordingly. In other words, companies will have to pay an increasingly higher price for increasingly more data. Therefore, the estimated growth of unstructured data cannot be sustained by human labor alone. Many companies are developing new, less labor-intensive methods to label data, but removing the human from the loop seems still far-fetched. Instead, we should ask ourselves whether companies do need all this data.
The New Data Pipeline
Within the current rush for data, collecting high-quality and unbiased data does not seem to have been the objective. Indeed, most data scientists know very well the struggle of preparing or “wrangling with” the data, which takes up to 80% of their time. Given the non-insignificant salary of data scientists, you may wonder why they spend so much time on clerical work. The answer is not that cleaning data is fun, but instead that it is necessary. Indeed, a model's performance – be it accuracy, generalizability, or false negatives – can only be as good as the data it gets fed. Therefore, deep learning models do not only require Big Data, but also Smart Data. Data that is relevant, unbiased, and varied.
To provide both Big and Smart Data for their models, companies first collect as much data as feasible, then outsource the labor-intensive labeling process, and lastly have their data scientists clean the data. However, the current sequence is highly inefficient: The fact that labeling takes place before cleaning means that companies spend lots of money to label data that they end up not using. Then, by identifying and removing biased, redundant, and noisy data before the labeling process takes place, companies can save money and time alongside the whole data pipeline.
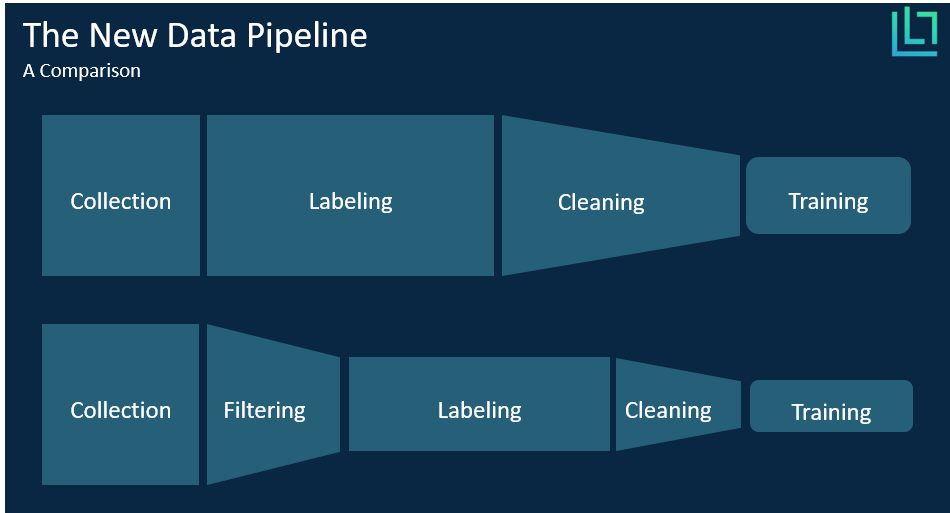
Nevertheless, cleaning the data before labeling is difficult. The issue is that without labels, one does not know what data has been collected. However, a relatively new and still unexplored branch of machine learning called self-supervised (or unsupervised) learning tackles specifically this problem. Self-supervised models can learn patterns from the data without the need to know the ground truth (i.e. labels). Among the many advantages of self-supervised learning, the identification of clusters, redundancies, and biases within unstructured data is paramount. Indeed, this property of self-supervised learning can be used to automatically clean the data, or more figuratively, filter it.
Therefore, by working directly on unstructured data, companies can avoid labeling costs and have their data scientists focus more on model development. Consequently, data filtering has a positive impact alongside the whole data pipeline and decreases the environmental impact of deep learning.
A Case for Self-supervised Learning
To show an example of the effectiveness of data filtering, we applied LightlyOne coreset subsampling method (which is based on self-supervised learning) on the KITTI dataset, a well-known benchmark for car-like objects detection. We compare it with random subsampling, which (astonishingly) is cur-
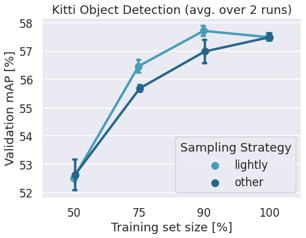
rently one of the most applied methods to filter unstructured data. As seen in the results plotted in Figure 4, LightlyOne’s self-supervised subsampling method beat the random method. But even more interesting, we were able to train a better model by utilizing only 90% of the dataset.
To conclude, the growth of unstructured data is currently not sustainable. From a labor and computing power perspective, deep learning is ethically questionable and not environment-friendly. Moreover, this condition will only worsen if not acted upon. Thus, companies must address this issue by rethinking their current data pipeline and deploy self-supervised solutions for data filtering.